Destacado
Transmission Control in NB-IoT With Model-Based Reinforcement Learning
In Narrowband Internet of Things (NB-IoT), the control of uplink transmissions is a complex task involving device scheduling, resource allocation in the carrier, and the configuration of link-adaptation parameters. Existing heuristic proposals partially address the problem, but reinforcement learning (RL) seems to be the most effective approach a priori, given its success in similar control problems. However, the low sample efficiency of conventional (model-free) RL algorithms is an important limitation for their deployment in real systems. During their initial learning stages, RL agents need to explore the policy space selecting actions that are, in general, highly ineffective. In an NB-IoT access network this implies a disproportionate increase in transmission delays. In this paper, we make two contributions to enable the adoption of RL in NB-IoT: first, we present a multi-agent architecture based on the principle of task division. Second, we propose a new model-based RL algorithm for link adaptation characterized by its high sample efficiency. The combination of these two strategies results in an algorithm that, during the learning phase, is able to maintain the transmission delay in the order of hundreds of milliseconds, whereas model-free RL algorithms cause delays of up to several seconds. This allows our approach to be deployed, without prior training, in an operating NB-IoT network and learn to control it efficiently without degrading its performance.
Autores
Alcaraz, J.J., Losilla, F., Gonzalez-Castaño, F.J.
Revista
IEEE Access
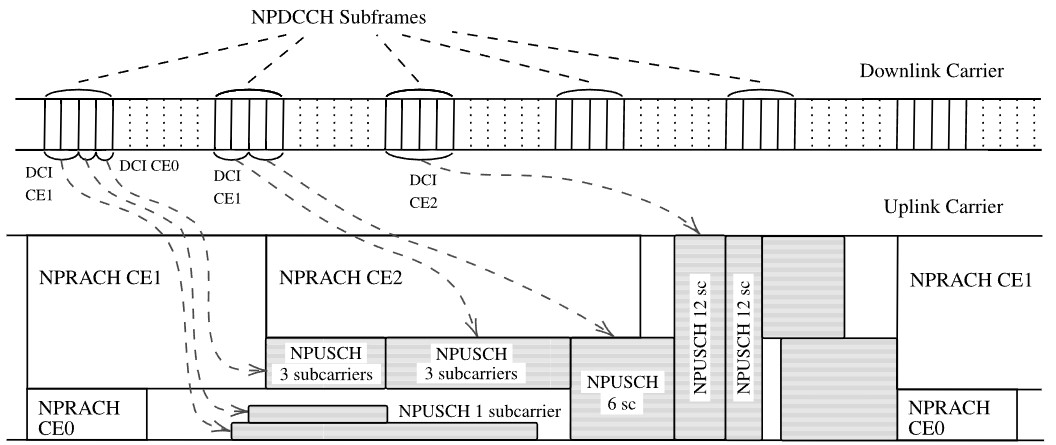
Publicado
9 de junio de 2023