Destacado
Model-Based Reinforcement Learning with Kernels for Resource Allocation in RAN Slices
This paper addresses the dynamic allocation of RAN resources among network slices, aiming at maximizing resource efficiency while assuring the fulfillment of the service level agreements (SLAs) for each slice. It is a challenging stochastic control problem, since slices are characterized by multiple random variables and several objectives must be managed in parallel. Moreover, coexisting slices can have different descriptors and behaviors according to their type of service (e.g., enhanced mobile broadband, eMBB, or massive machine type communication, mMTC). Most of the existing proposals for this problem use a model-free RL (MFRL) strategy. The main drawback of MFRL algorithms is their low sample efficiency which, in an online learning scenario (i.e., when the agents learn on an operating network), may lead to long periods of resource over-provisioning and frequent SLA violations. To overcome this limitation, we follow a model-based RL (MBRL) approach built upon a novel modeling strategy that comprises a kernel-based classifier and a self-assessment mechanism. In numerical experiments, our proposal, referred to as kernel-based RL (KBRL), clearly outperforms state-of-the-art RL algorithms in terms of SLA fulfillment, resource efficiency, and computational overhead.
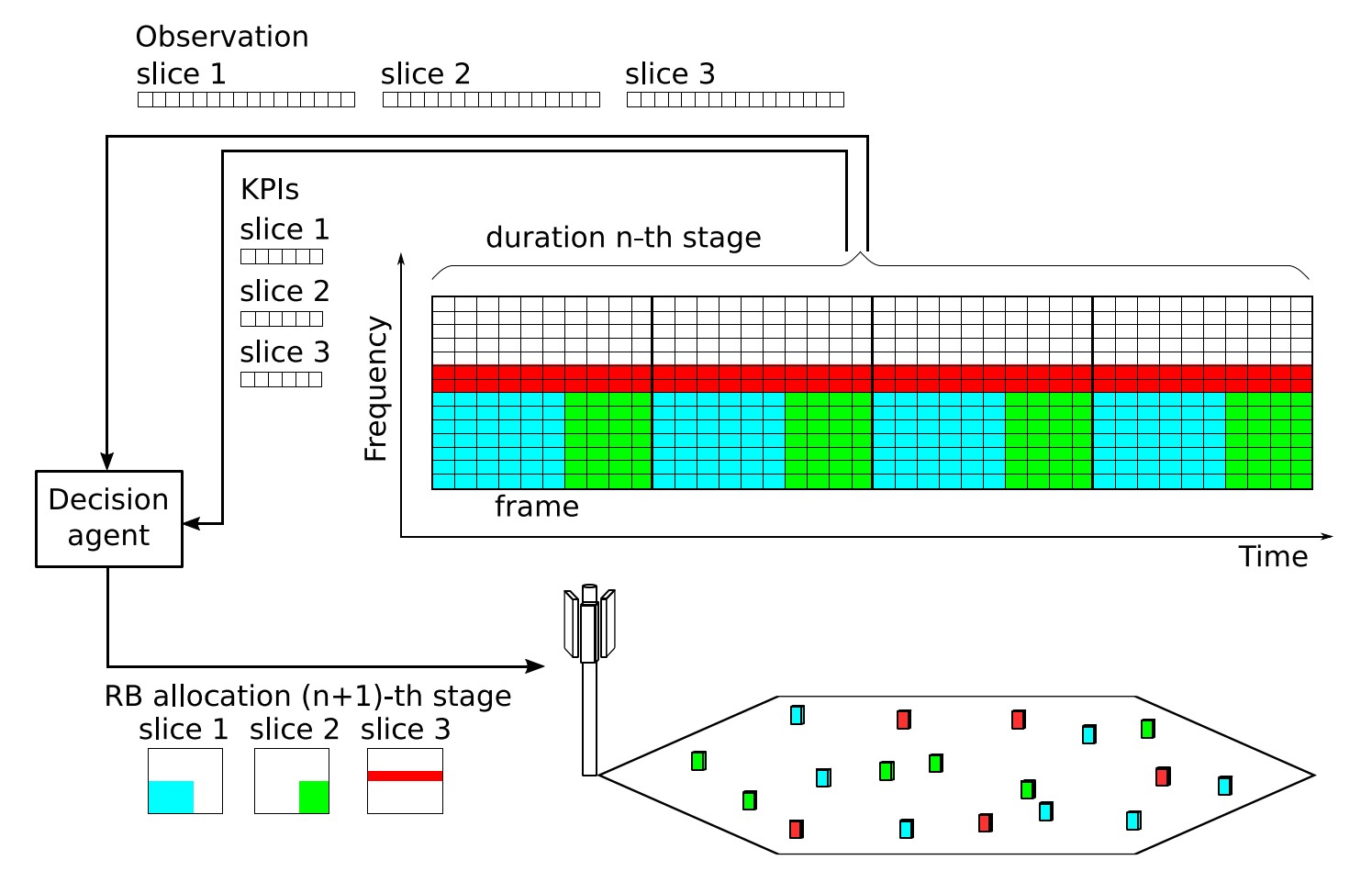
Autores
Alcaraz, J.J., Losilla, F., Zanella, A., Zorzi, M.
Revista
IEEE Transactions on Wireless Communications
Publicado
9 de agosto de 2022